Brain-Inspired Chip Design Stands the Test of Time
By Miranda Volborth
Ten years ago, three destined-to-be Duke engineers rethought the way data could be stored and computed
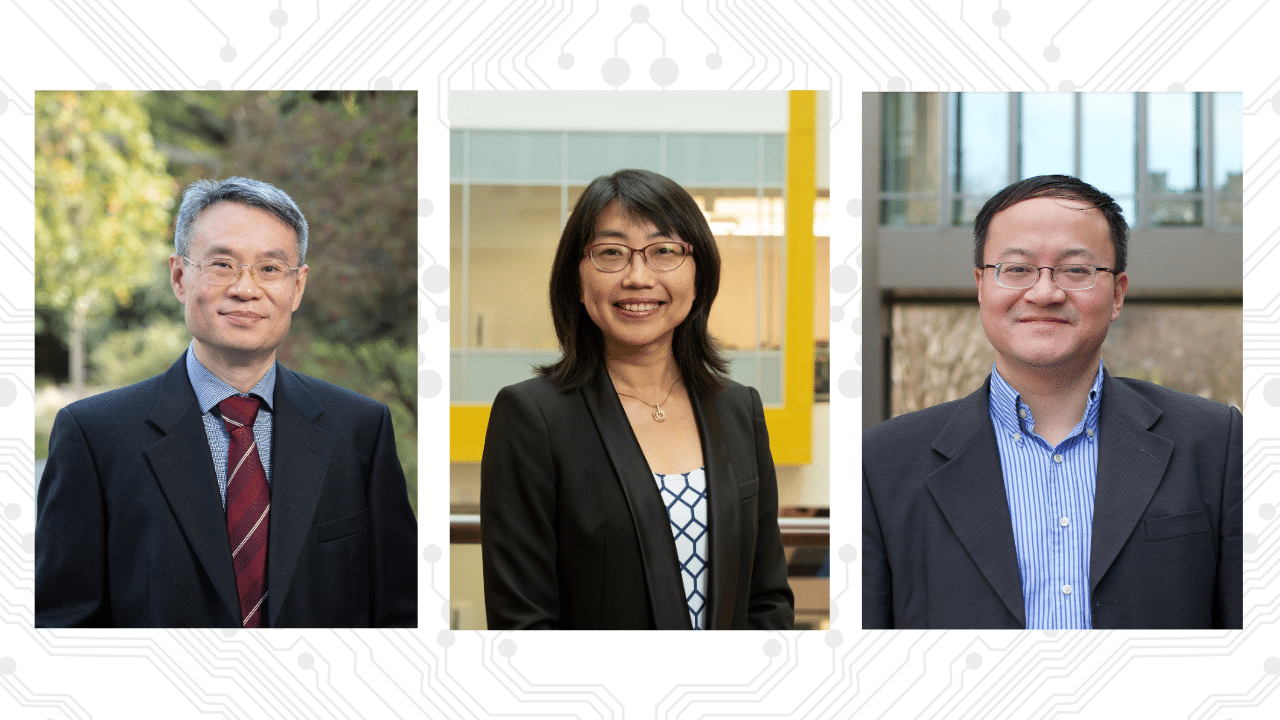
The amount of time and energy spent shuttling data from one location to another—writing results, storing them, and retrieving them to perform calculations—consumes the major share of a computer’s time and energy. In fact, the data traffic jams that occur as information flows from point to point are known collectively as the “memory wall.”
Ten years ago, machine learning applications were starting to take off, and they demanded even more resources than ever before. Entirely new types of computer architectures were needed to scale the growing memory wall.
One of these new architectures, neuromorphic computing, was inspired by the way the human brain sorts and classifies information. Its continuing evolution is enabled by non-volatile memristors, which retain memory without needing to be powered on.
A design methodology proposed by three engineers who have since joined the Duke electrical and computer engineering faculty—Yiran Chen, Hai Li and Xin Li —along with collaborators Beiye Liu, Tingwen Huang, Qing Wu and Mark Barnell, detailed the kind of memristor arrays that could best accelerate neural networks and perhaps eventually enable neural networks on a single chip. Their scheme significantly shrank memristor-based AI accelerators’ footprints by more than a quarter while improving computing accuracy.
“Almost every semiconductor company now has a research arm in this area. Many startups have been founded around this technology, too.”
Yiran Chen
John Cocke Distinguished Professor of Electrical and Computer Engineering
Now their paper, “Reduction and IR-drop Compensations Techniques for Reliable Neuromorphic Computing Systems,” has been awarded a Test of Time Award from the IEEE Council on Electronic Design Automation (IEEE CEDA). These 10-year retrospective awards are given to papers judged to be the most influential on research and industry practice in computer-aided design of integrated circuits.
In the decade since the paper was published, Chen and Li have continued to focus on chip design, refining and advancing strategies to make computing more efficient and reliable. Their novel computer architectures will advance machine learning, but machine learning is also being used to design the architectures; how large an array is needed, what shape it should be and how many resistive random-access memory cells it requires to accomplish a given task are all tasks that machine learning is well-equipped to tackle.
“At the time this paper was published, people had barely started accelerating the neural network. Since then, people have come up with many different ways to accelerate computation through design,” said Chen, the John Cocke Distinguished Professor of Electrical and Computer Engineering. “And it sparked substantial investment from industry. Almost every semiconductor company now has a research arm in this area and they are trying to commercialize—or have already commercialized— products that hardware designers can use. Many startups have been founded around this technology, too.”
Offshoots of the idea have even grown into entirely new branches. A former PhD student in Chen’s lab, when he was at the University of Pittsburgh, accepted a Best Paper of 2023 award from the International Conference on Computer-Aided Design at the same time Chen received his Test of Time Award.
The former student, Wujie Wen, is now an associate professor at North Carolina State University and works on processing-in-memory, or PIM, which approaches the challenge of reliability in a new way, by deploying non-volatile memory technology on crossbar arrays in a way that circumvents the need for data to be moved at all.
“It is based on the idea of memristor arrays, but the technology and even the concept have evolved into a whole new field,” said Chen. “I enjoyed sharing the stage with Wujie to accept these two different awards together.”
Explore Duke ECE
Duke ECE is among the top AI and machine learning research enterprises in the world.